🔥 Devs Are Using These AI Tools to Code 10x Faster in 2025 — Why Aren’t You?
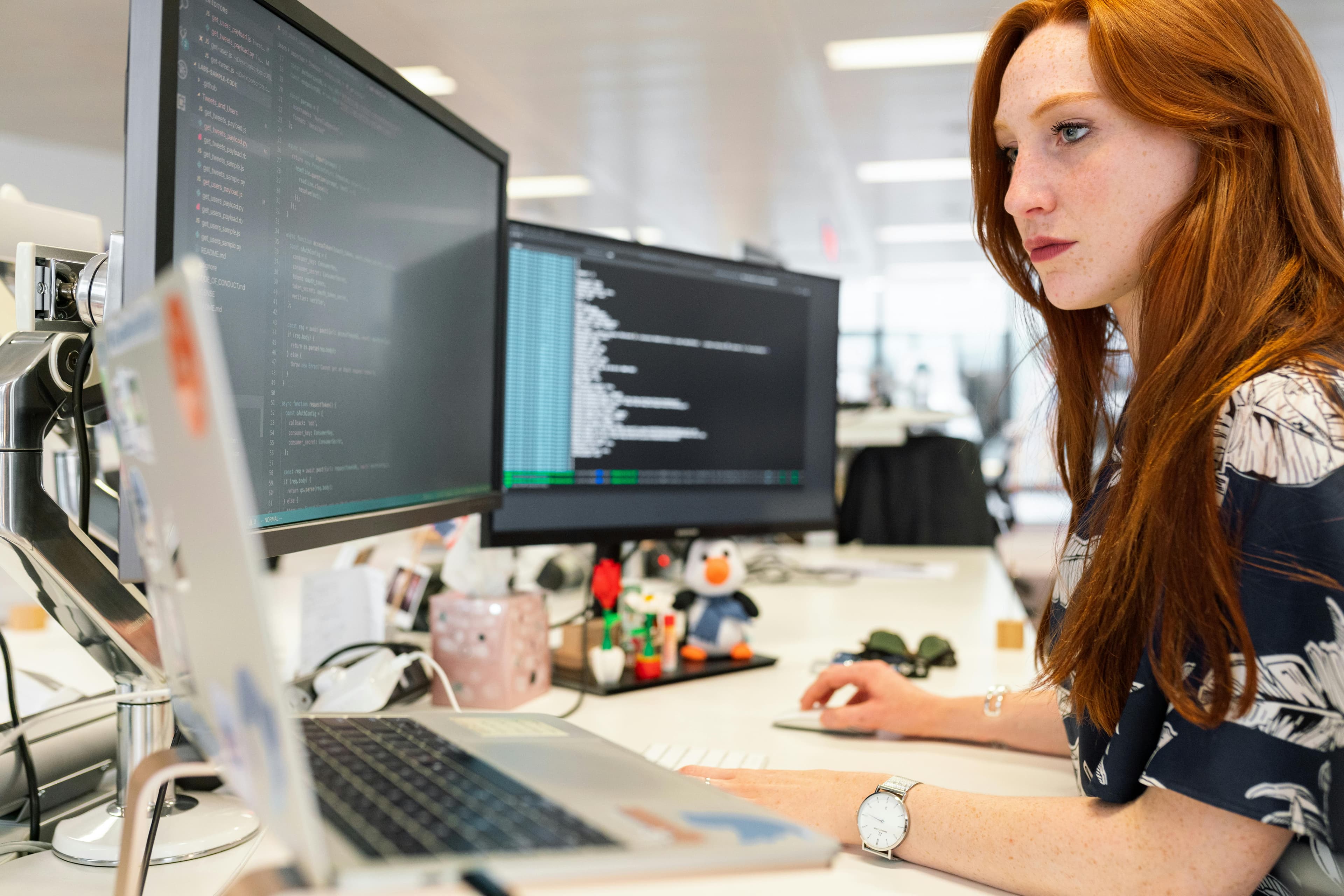
From Copilot to Codeium and beyond — discover how devs are shipping 40% faster, writing fewer bugs, and building smarter in 2025 using AI. This guide shows you the best tools, real-world use cases, and how to start right now.
TL;DR – AI is no longer a side‑kick in software projects; in 2025 it’s the co‑pilot, QA engineer, tech writer, and project planner rolled into one. In this guide you’ll learn how AI is reshaping every stage of the development lifecycle, which tools you should know, where they shine (and where they still stumble), and how to adopt them responsibly.
1. Why 2025 Is a Breakthrough Year for AI and Software Development
Three converging trends make 2025 the inflection point:
- Ubiquity of large‑context coding models. OpenAI’s GPT‑4o, Google’s Gemini Code Assist, and Meta’s Code LLaMA 400B can now ingest entire repos, turning “explain this legacy module” into a five‑second task.
- Enterprise‑grade copilots. GitHub Copilot Enterprise, Codeium’s Windsurf platform, and Tabnine Enterprise integrate with private code bases, security scanners, and CI/CD, giving teams AI assistants that understand their proprietary patterns.
- Proven ROI. Companies using AI assistants see 30‑40 % productivity gains according to Windsurf (Codeium) CEO Varun Mohan, yet they’re increasing headcount because the economic return per engineer jumps dramatically.
With budgets tighter and release cycles shorter, ignoring AI is no longer optional.
2. The 2025 AI Toolbelt — What’s in It and When to Reach for Each Tool
2.1 AI Code Generation & Autocomplete
Tool | What It Excels At | Quick Win |
---|---|---|
GitHub Copilot | Autocompletes multi‑line logic in 20+ languages; tight IDE integration | Scaffold REST endpoints in Express or FastAPI in minutes |
Codeium / Windsurf | Context‑aware suggestions plus a chat pane trained on your repo | Convert imperative Python to idiomatic Rust |
Tabnine | Privacy‑focused autocomplete that can stay 100 % on‑prem | Safe option for finance or healthcare code bases |
Cursor & Aider | Lightweight, CLI‑first assistants that edit files in‑place | Refactor a React component from class to hooks |
Teams report shaving 1.3Ă— to 2Ă— off boilerplate time with these generators.
2.2 AI‑Powered Debugging & Error Fixing
- ChatGPT Code Interpreter – paste an error trace, get stack‑aware fixes.
- DeepCode AI (now Snyk Code) – performs static analysis, pinpoints security flaws, and proposes one‑click fixes.
- Gemini Code Assist – understands Android stack traces and suggests patch diffs.
Pro tip: Pair your debugger copilot with unit‑test generation (see § 2.3) so each fix ships with a safety net.
2.3 Automated Testing & Quality Assurance
- Testim Autonomous – records UI interactions and turns them into self‑healing end‑to‑end tests.
- Waydev’s AI QA suite – mines commit history, surfaces flaky tests, and predicts hot‑spot files before they break prod.
- Copilot workflows – generate parameterized Jest or PyTest cases from docstrings.
Teams that integrate AI‑driven test generation early report 40 % fewer escaped defects and noticeably happier QA engineers.
2.4 Documentation & Knowledge Bots
- Mintlify Doc Writer – scans a code file and drafts human‑readable API docs.
- ChatGPT voice‑note → README – dictate a high‑level architecture overview, get a Markdown diagram back.
- Codeium Chat – answers “how does our caching layer work?” directly from your private repo.
2.5 Project Estimation & Planning
- Jira AI – converts epics into groomed user stories, estimates with Monte‑Carlo sims.
- Azure DevOps Copilot – auto‑generates sprint tasks from PR descriptions.
- Linear AI – predicts cycle time based on historical velocity.
By letting AI handle the busy‑work of estimation, PMs focus on risk, scope, and stakeholder alignment.
2.6 Security & Code Review
Security scanners now speak natural language:
- Snyk Code (DeepCode AI) – hybrid symbolic + generative AI that flags vulnerabilities and explains why a fix matters.
- SonarLint AI – inline secure‑coding suggestions in VS Code.
- GitHub Advanced Security + Copilot – surfaces secrets, unsafe dependencies, and proposes patch PRs you can merge in one click.
3. Real‑World Examples of AI at Work
3.1 Leveling‑Up Junior Devs
Meet Maya, a recent boot‑camp grad at a fintech startup. On her first ticket—adding pagination to a legacy Django view—she feeds the current file to ChatGPT with the prompt “Refactor to class‑based view and add cursor pagination (PostgreSQL). Include unit tests.” The assistant returns clean, idiomatic code plus tests. A senior reviews it in minutes. Maya learns best practices by example, not trial‑and‑error.
3.2 Lightning‑Speed MVPs for Startups
Seed‑stage founder Ravi builds an AI‑powered note‑taking SaaS. In week 1 Copilot scaffolds the React/Next.js UI; week 2 Codeium helps generate GraphQL resolvers; by week 3 Testim AI nails E2E tests. He hits demo‑day with a polished prototype that would’ve taken months with a two‑person crew.
3.3 Enterprise Governance That Finally Scales
A Fortune 100 bank integrates DeepCode AI into its monorepo. The tool catches insecure crypto usage before code reviews, and the bank’s average CVSS score for newly merged code drops from 8.1 to 4.2 in one quarter.
4. Benefits of Pairing AI and Software Development
Benefit | Why It Matters |
---|---|
Speed | Ship features 30–40 % faster on average |
Quality | AI linters catch edge cases humans miss, reducing prod bugs |
Onboarding | New hires query the codebase in plain English instead of hunting docs |
Consistency | Style‑guides, commit messages, and tests follow autogenerated templates |
Developer Joy | Less boilerplate means more time for creative problem‑solving |
5. Limitations & Ethical Considerations
- Hallucinations & subtle bugs. Language models may return syntactically correct but logically wrong code—always review before merging.
- Security & IP leakage. Sending proprietary code to third‑party APIs can violate compliance. Prefer on‑prem or enterprise plans with encrypted storage.
- License uncertainty. Generated snippets may contain GPL‑licensed patterns; use scanners such as FOSSA or Socket.dev.
- Skill atrophy. Over‑reliance can dull problem‑solving muscles. Use AI to amplify, not replace, reasoning.
- Bias & ethics. Training data skews can perpetuate insecure or discriminatory patterns. Static analysis plus human review remains essential.
6. Best Practices for Adopting AI Responsibly
- Start small, measure, iterate. Pilot in a single repo; track cycle time, bug rates, and sentiment.
- Establish a policy. Define which data can be shared with external APIs and require opt‑in for sensitive modules.
- Bake AI into CI/CD. Failing a DeepCode security check blocks the merge; passing regenerates docs automatically.
- Pair programming 2.0. Encourage devs to talk to their copilot while keeping a human reviewer in the loop.
- Invest in upskilling. Teach prompt engineering, AI ethics, and the craft of clean code.
7. FAQ — Quick Answers for Curious Beginners
Q1. Do I need to learn machine learning to use AI coding tools?
No. Most assistants integrate as VS Code extensions or CLI tools. You interact via plain‑language prompts.
Q2. Is AI‑generated code production‑ready?
Often, but never trust blindly. Treat AI output like code from a junior engineer: review, test, and secure before shipping.
Q3. Will AI take developer jobs?
Evidence suggests the opposite: productivity gains increase ROI, so companies hire more engineers, not fewer.
Q4. Which tool should I start with?
Begin with GitHub Copilot or Codeium—they’re plug‑and‑play, language‑agnostic, and have generous free tiers.
Q5. How do I keep private code private?
Use on‑prem versions (Tabnine Enterprise, Codeium Self‑Hosted) or tools that offer encrypted context windows.
8. The Road Ahead — Embrace the Augmented Future
AI won’t write the next breakthrough algorithm on its own—you will. But 2025’s toolbelt means you’ll spend less time wrangling boilerplate and more time architecting features that matter. The developers who thrive will be those who:
- Understand when to trust the machine and when to dig deeper.
- Treat AI as a collaborative colleague, not a magic wand.
- Keep learning: prompt engineering, ethics, and the craft of clean code.
So fire up your favorite IDE, invite your shiny new copilot into the project, and build something incredible. The future of AI and software development isn’t about humans vs. machines—it’s about humans plus machines, coding side‑by‑side to push the boundaries of what software can do.
Happy building! 🚀
Related Posts
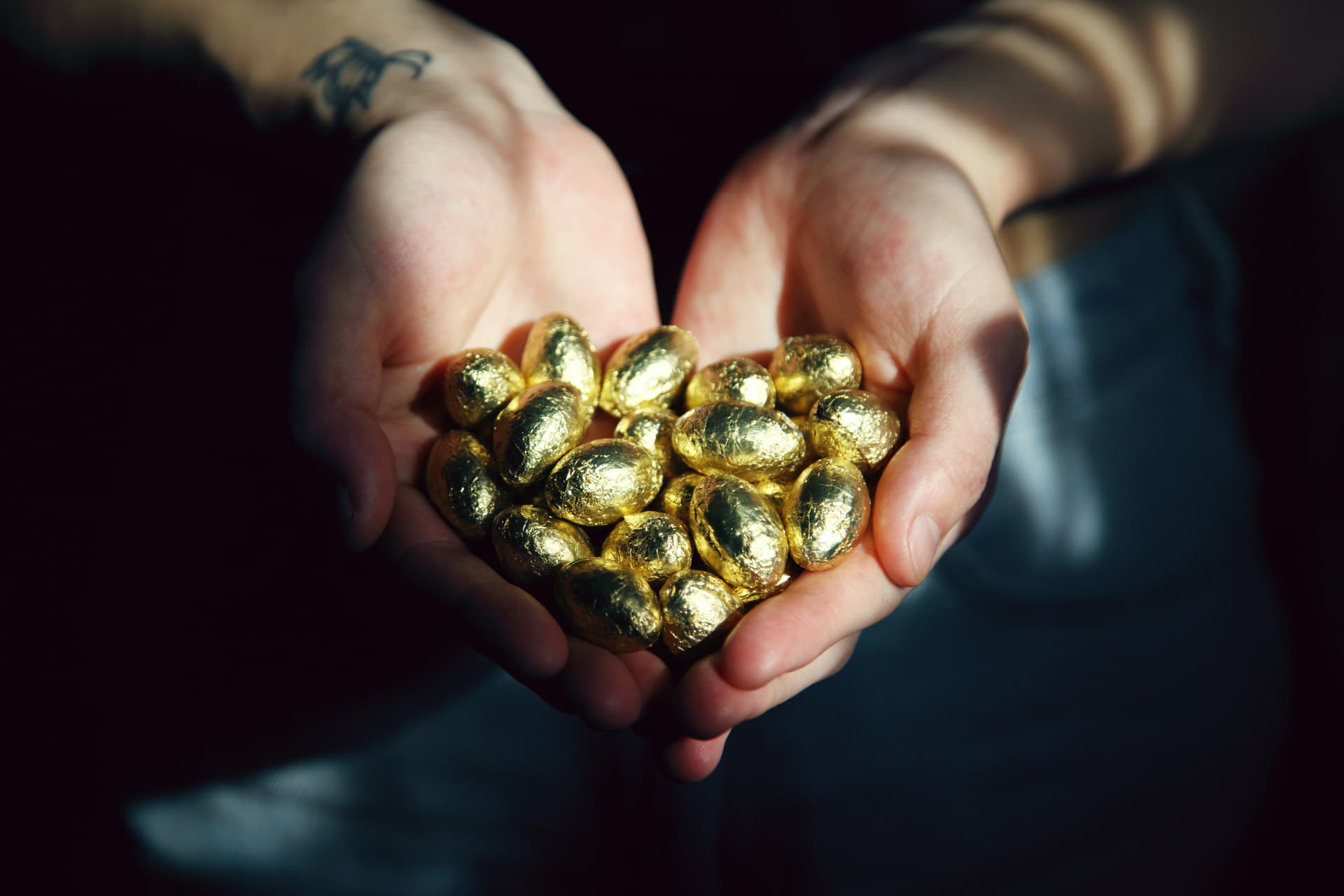
The 17 Principles of Creating Wealth That Changed My Life (Most People Ignore #5)
Building wealth isn’t just about money — it’s about mindset. These 17 powerful principles helped me go from broke to financially secure, and they can work for anyone willing to try.
Read Full Story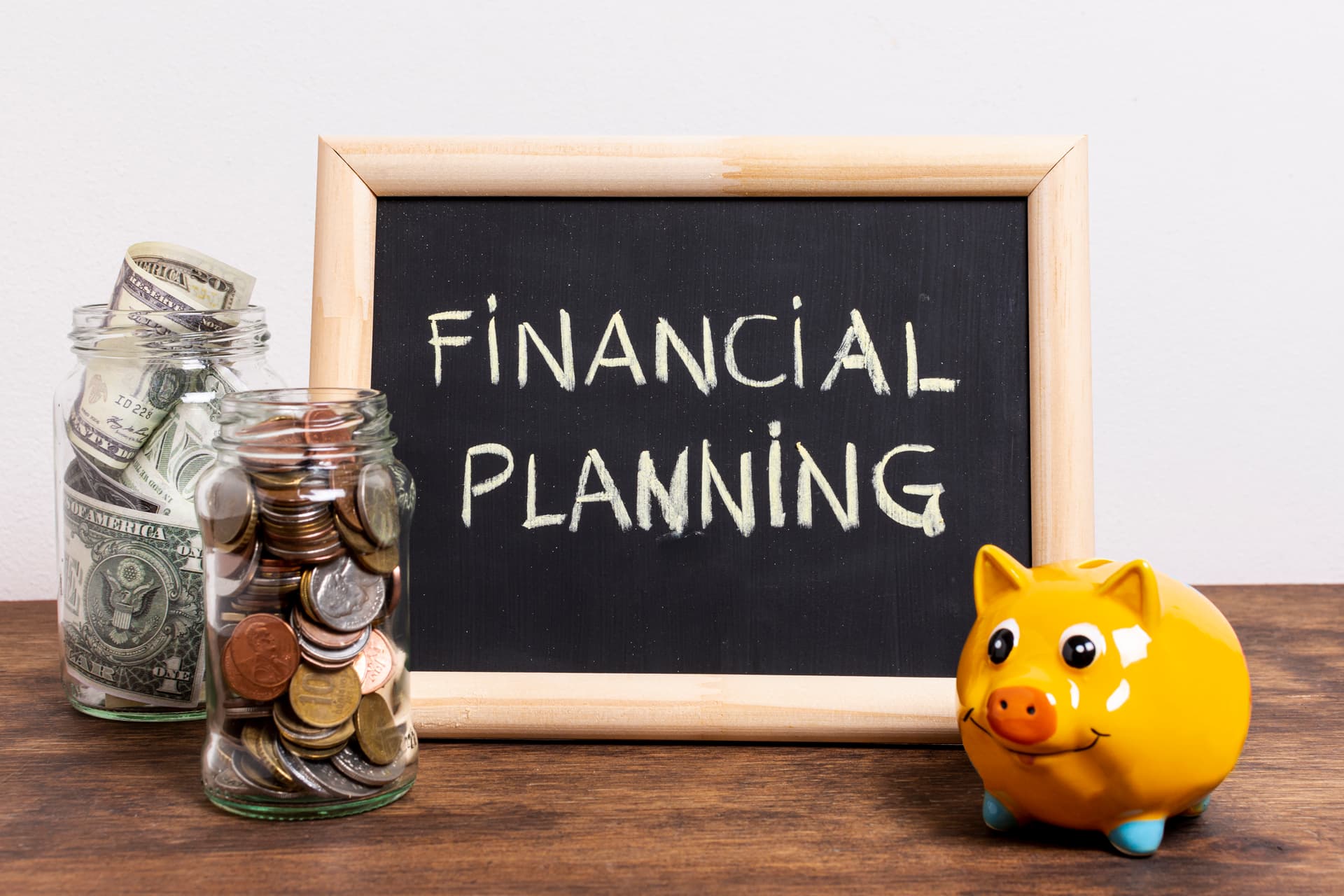
I Built an Emergency Fund From $0 in Just 30 Days — Here’s the Exact Plan I Followed
Broke, stressed, and unprepared — that was me a month ago. But in just 30 days, I went from zero savings to building my first emergency fund. Here’s the simple plan that actually worked.
Read Full Story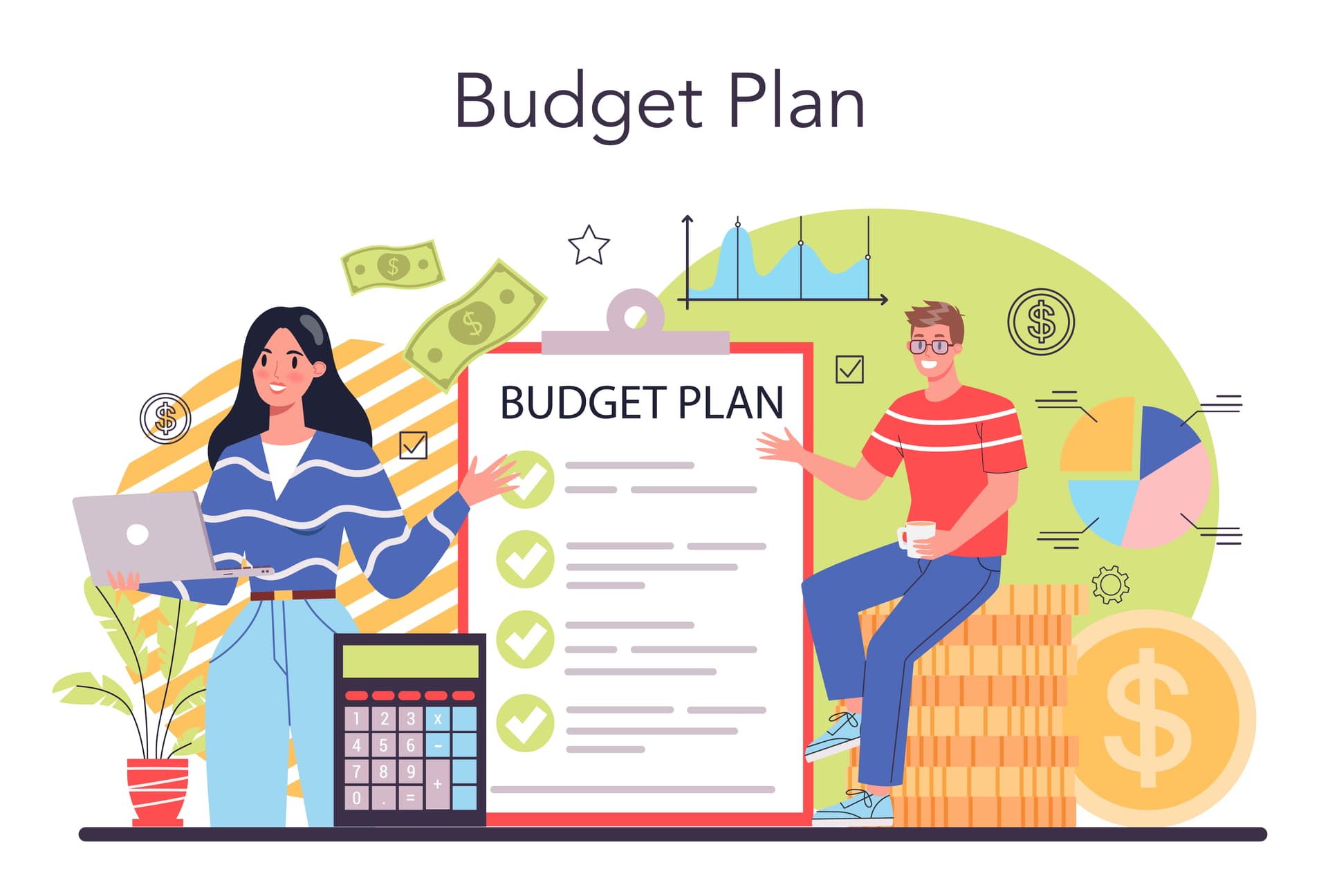
I Let AI Manage My Budget for 30 Days — Here’s How Much I Actually Saved
I used to struggle with budgeting — until I handed it all over to AI. Here’s what happened after 30 days of letting artificial intelligence manage my money (and how much I actually saved).
Read Full StoryAdd a Comment
Recent Comments
Loading comments...